Configuration#
Since fish diffusion supports various modules, writing a good config file is essential. There are many examples in the configs folder.
To begin with, you should create a config file exp_xxxxxx.py
in the configs
folder with the following code:
_base_ = [
"./_base_/archs/diff_svc_v2.py", # Using Diff SVC v2 architecture
"./_base_/trainers/base.py", # Use default trainer (with DDP + fp16)
"./_base_/schedulers/warmup_cosine.py", # Use Cosine Learning Rate Scheulder
"./_base_/datasets/audio_folder.py", # Use the default dataset and dataloader
]
Preprocessing#
Preprocessing config currently includes two components: text_features_extractor
and pitch_extractor.
Stable feature extractors are HubertSoft
, ChineseHubertSoft
, and ContentVec
. Although there is a Chinese
in the second one, it works on multilingual and outperforms the vanilla one.
To extract the pitch, you can choose ParselMouthPitchExtractor
or CrepePitchExtractor
. ParselMouth is good enough in most cases, and it’s literally 100x faster than Crepe. However, in some edge cases, Crepe is more robust and stable. For more results, see Pitch Extractors.
# HubertSoft Example
preprocessing = dict(
text_features_extractor=dict(
type="HubertSoft",
),
pitch_extractor=dict(
type="ParselMouthPitchExtractor",
),
)
# ChineseHubertSoft Example
text_features_extractor=dict(
type="ChineseHubertSoft",
pretrained=True, # Use Lengyue's Chinese Hubert Soft Model
gate_size=25, # Controlling how much information can be kept. Too large will lead to information leaking.
),
Note: You need to rerun the preprocessing command after you change the preprocessing config.
Dataset#
Be default, ./_base_/datasets/naive_svc.py
will try to load data from dataset/train
and dataset/valid
. However, if you want to train a multi speakers model, you should refer to svc_hubert_soft_multi_speakers.py
. For example:
dataset = dict(
train=dict(
_delete_=True, # Delete the default train dataset
type="ConcatDataset", # You want to contact multiple datasets
datasets=[
dict(
type="NaiveSVCDataset",
path="dataset/speaker_0", # First speaker's data folder
speaker_id=0, # Dataset for the first speaker
),
dict(
type="NaiveSVCDataset",
path="dataset/speaker_1",
speaker_id=1,
),
],
# Are there any other ways to do this?
collate_fn=NaiveSVCDataset.collate_fn,
),
valid=dict(
type="NaiveSVCDataset", # Only use one speaker to validate
path="dataset/valid",
speaker_id=0,
),
)
model = dict(
speaker_encoder=dict(
input_size=2, # 2 speakers
),
)
Since this is a Python script, you can load dataset from any folder you like.
But… What if I have 100+ speakers? I can’t setup manually and I don’t know how to write Python code :(
Don’t worry. You can use the following folder structure and scripts:
dataset
├───train
│ ├───speaker0 # Train data for speaker 0
│ | └───xxx1-xxx1.wav
│ └───speaker1
│ └───xxx1-xxx1.wav
└───valid
├───speaker0 # Test data for speaker 0. You can pick some from other speakers
| └───xxx1-xxx1.wav
└───speaker1
└───xxx1-xxx1.wav
from fish_diffusion.datasets.utils import get_speaker_map_from_subfolder, get_datasets_from_subfolder
speaker_mapping = {}
speaker_mapping = get_speaker_map_from_subfolder("dataset/train", speaker_mapping) # Update speaker_mapping using subfolders in `dataset/train`.
# This will update speaker_mapping to {'speaker0': 0, 'speaker': 1}
train_datasets = get_datasets_from_subfolder("NaiveSVCDataset", "dataset/train", speaker_mapping) # Build datasets manually.
valid_datasets = get_datasets_from_subfolder("NaiveSVCDataset", "dataset/valid", speaker_mapping) # Build datasets manually.
dataset = dict(
train=dict(
_delete_=True, # Delete the default train dataset
type="ConcatDataset", # You want to contact multiple datasets
datasets=train_datasets,
# Are there any other ways to do this?
collate_fn=NaiveSVCDataset.collate_fn,
),
valid=dict(
_delete_=True, # Delete the default train dataset
type="ConcatDataset", # You want to contact multiple datasets
datasets=valid_datasets,
# Are there any other ways to do this?
collate_fn=NaiveSVCDataset.collate_fn,
),
)
model = dict(
speaker_encoder=dict(
input_size=len(speaker_mapping),
),
)
Data Augmentation#
To enable data augmentation, you can reference to the following config:
preprocessing = dict(
...
augmentations=[
dict(
type="FixedPitchShifting",
key_shifts=[-5., 5.],
probability=0.75,
),
dict(
type="RandomPitchShifting",
key_shifts=[-5., 5.],
probability=1.5,
),
dict(
type="RandomTimeStretching",
factors=[0.8, 1.2],
probability=0.75,
),
]
)
After editing the config, you need to rerun the preprocessing command.
Note: You should not use
RandomPitchShifting
andRandomTimeStretching
at the same time.
RandomTimeStretching is not tested yet (as of 2023-03-03).
Appendix: Pitch Extractors#
Currently, this repo supports ParselMouth, Crepe, Harvest, and Dio. However, we recommend only using Crepe and ParselMouth.
If your dataset is small and you want it to be more robust, Crepe is probably good for you.
Otherwise, you should use ParselMouth since it is much faster.
Here are some comparisons:
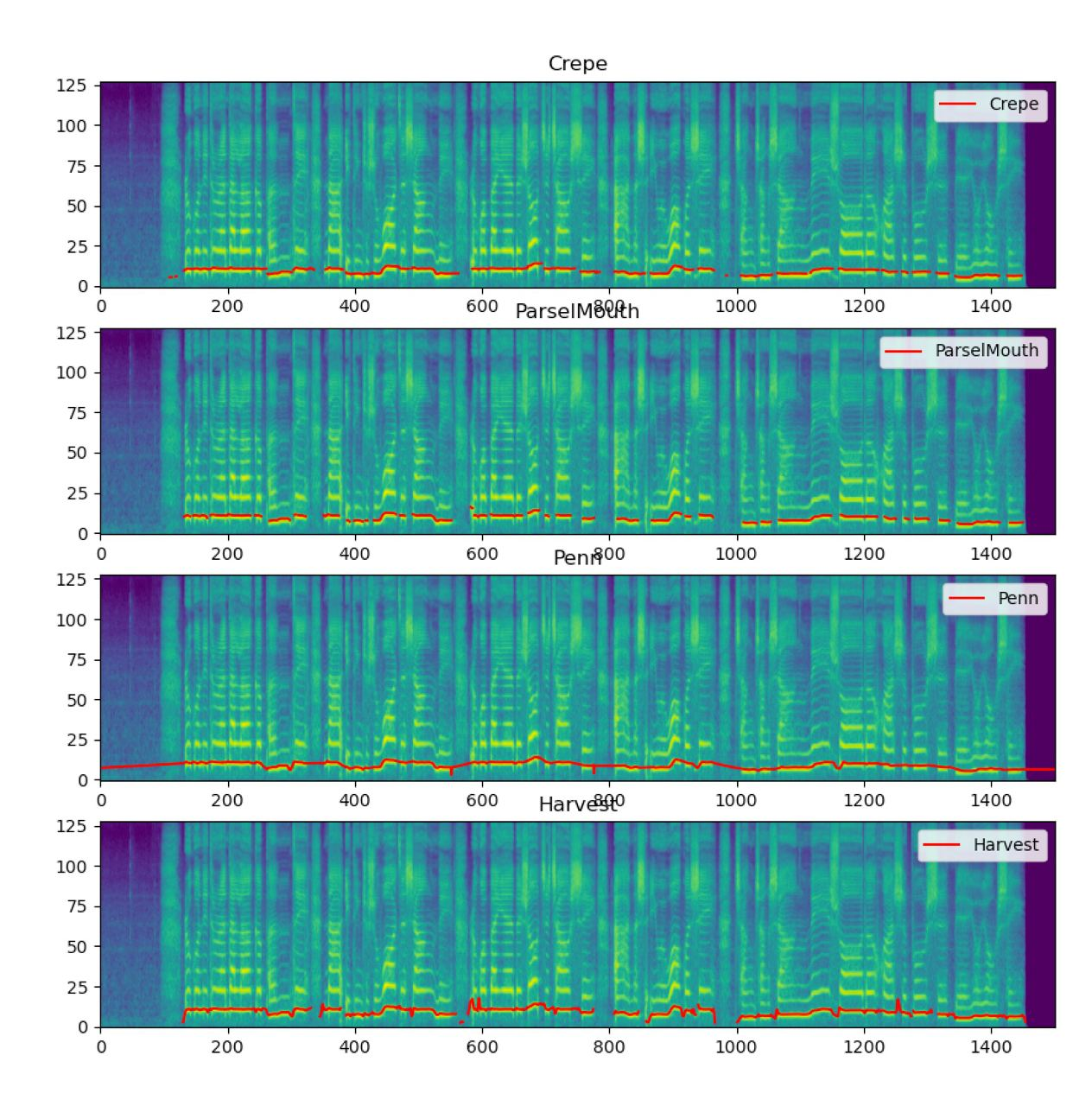
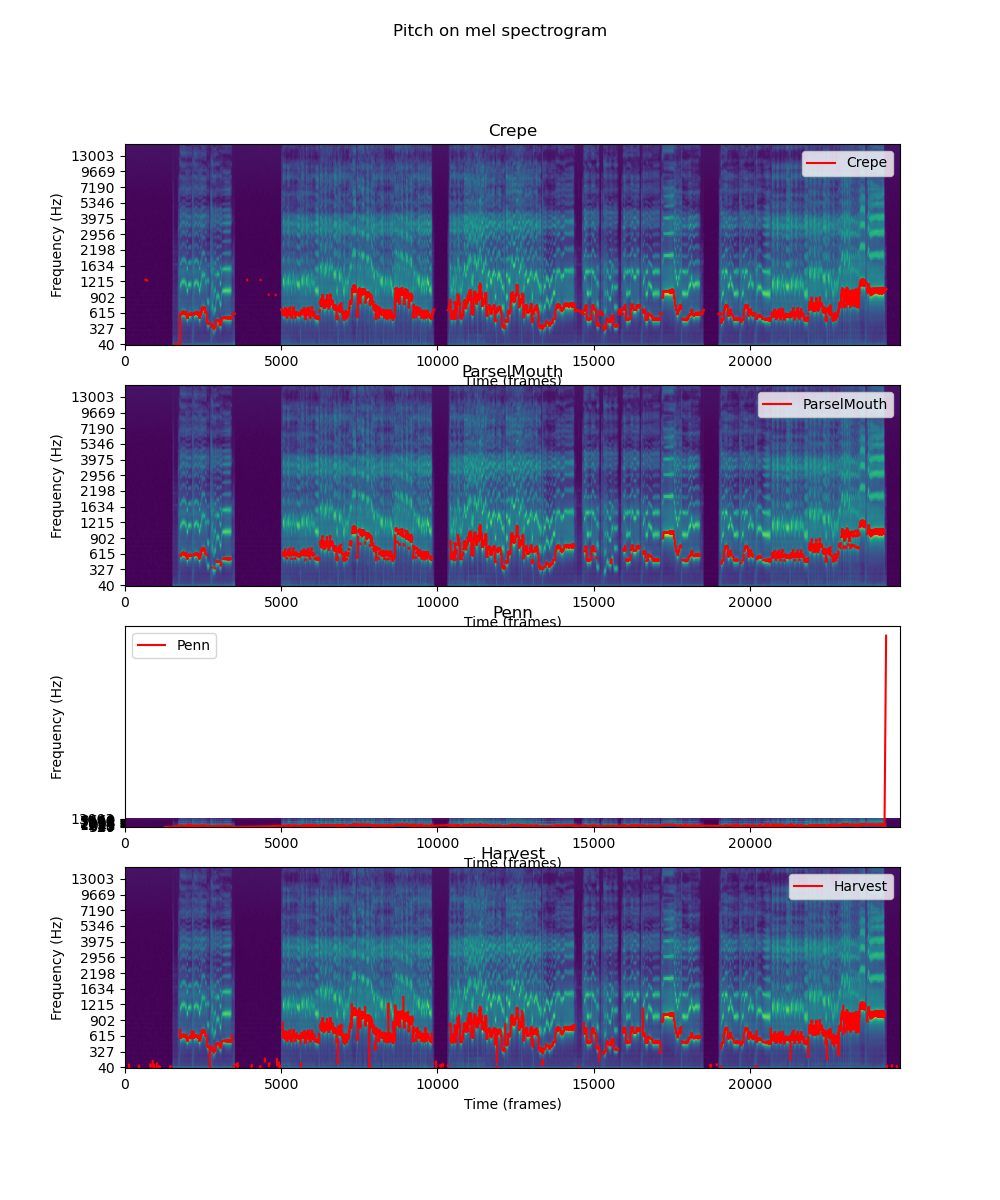